Posted By: HGG Advances
Each month, the editors of Human Genetics and Genomics Advances interview an early-career researcher who has published work in the journal. This month we check in with Diptavo Dutta (@Diptavo) to discuss his paper “Subset-based method for cross-tissue transcriptome-wide association studies improves power and interpretability”.
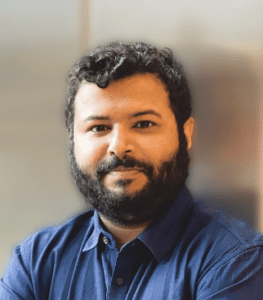
HGGA: What motivated you to start working on this project?
DD: The scientific motivation of this work was to investigate, for diseases, whether there are tissues beyond the obvious candidates where genes might manifest associations, and if so, can we interpret such findings from an etiologic viewpoint. The statistical method is a broad extension of ASSET, Association analysis based on subsets, (AJHG, 2012) and GAUSS, Gene set analysis Association Using Sparse Signals, (AJHG, 2021). However, the application to meta-analyze gene-based association across tissues while picking the best subset of tissues might identify tissues beyond the likely suspects, thus providing newer insights into disease development and progression.
HGGA: What about this paper/project most excites you?
DD: In general, meta-analysis methods have (almost) always aggregated information across multiple studies/contexts. However, I believe that the idea of being able to choose the best or optimal subset of studies/contexts that collectively maximize the association has a unique merit from a statistical standpoint. This enables us to look beyond the obvious disease-tissue/context pairs and interrogate other contexts on whether they manifest any (possibly weaker) association with the disease. Such signatures can potentially open the door to understanding how risk factors influence disease risk or perhaps targets of gene-environment interaction.
HGGA: What do you hope is the impact of this work for the human genetics community?
DD: As we get more granular in terms of profiling molecular landscapes, the sample size almost always goes down due to current state of cost-effective profiling technologies. Analytical approaches that can boost statistical power while preserving granularity of information would be valuable to improve detection while providing better interpretation as well as etiological insights. We are already extending the work to cell-type-specific analysis, and I hope such approaches will be handy in single-cell studies in the future as well.
HGGA: What are some of the biggest challenges you’ve faced as a young scientist?
DD: There are several factors that I have faced while starting my career as a PI, one of them being the amount of logistical detailing which is a part and parcel of every independent scientist. That, coupled with the pressure of juggling simultaneously through several (> 5) projects with my trainees while keeping up with the current works in science and coming up with novel ideas seemed overwhelming at first. But fortunately, with a great support group at my current institution, as well as my trainees, I have been able to (sort of) stabilize myself from the initial jerk.
HGGA: And for fun, what is one of the most fascinating things in genetics you’ve learned about in the past year or so?
DD: I am curiously learning about how large-scale CRISPR knockout screens can inform disease biology. The technology is probably at a stage where it warrants thoughtful statistical methods, especially causal inferential techniques, to be devised.