Posted By: Kylee Spencer, PhD, Assistant Editor, AJHG
Each month, the editors of The American Journal of Human Genetics interview an author of a recently published paper. This month, we check in with Yosuke (@yk_tani) to discuss his recent paper, “Power of Inclusion: Enhancing polygenic prediction with admixed individuals.”
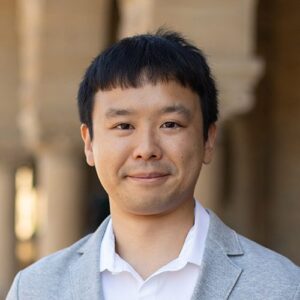
KS: What motivated you to start working on this project?
YT: As a researcher, it is important for me to think about this problem: what can you do to ensure the results and the benefits of your research are equally shared by everyone?
It has been widely recognized that polygenic scores (PGS) have limited transferability across populations. Like many other researchers in the field, I also observed the phenomenon many times in my previous research, and I wanted to address the problem as a computational scientist.
The challenges and opportunities seem twofold: datasets and computational techniques. On the dataset front, which I believe to be the primary solution to the problem, there are already many ongoing efforts. On the computational front, there have also been a number of great methodological innovations, such as approaches that consider multiple population groups when building predictive models from summary statistics from genome-wide association studies (GWAS) and ancestry-matched linkage disequilibrium (LD) reference panels.
I realized that a relatively small number of studies focus on PGS models for admixed individuals despite the fact that an increasing number of publications highlight the importance of considering the continuum of, rather than distinct groups of, genetic ancestry in human genetics research. The standard PGS procedure is not designed to consider genetic ancestry as a continuum because it requires researchers to assign individuals (or local genomic segments of individuals) to distinct (local) ancestry groups.
I hypothesized that it would be possible to overcome the technical limitations by using an alternative approach: PGS training directly on the individual-level data. Luckily, we have previously developed a method for such an approach, thanks to fruitful collaborations with colleagues in the statistics department. We set out to see if the direct inclusion of diverse individuals in PGS training would help mitigate the limited PGS portability problem.
KS: What about this paper/project most excites you?
YT: Don’t get me started! I am excited about the many aspects of our study, including the improved predictive performance of our inclusive PGS models, most notably in individuals of African ancestry, with an average improvement of 60%.
What is even more exciting to me is our proposal on the iPGS+refit strategy. Many existing PGS approaches model ancestry-specific genome-wide polygenic components while allowing the correlation of effect sizes across ancestry groups. On the other hand, our approach starts with one ancestry-shared component and adds ancestry-dependent components using a relatively small number of genetic loci. The reparameterization in our approach facilitates better interpretation for genomic loci with ancestry-dependent effects in the predictive models, and our results on hematological traits show its advantage in improving predictive performance.
KS: Thinking about the bigger picture, what implications do you see from this work for the larger human genetics community?
YT: Our work illuminates three major directions for future human genetics research.
First, our study underscores the importance of considering ancestry-shared genetic effects in common complex traits. We evaluated our approach, focusing on anthropometric and hematological traits. However, it does not mean those are the only traits that would benefit from the proposed approach. Clearly, as the research community, we should expand the analysis across a wide variety of phenotypes, including disease and non-disease traits, to see if inclusive modeling of the ancestry-shared genetic effects would be helpful.
Second, our work highlights the critical importance of considering the continuum of genetic ancestry. We focused on the PGS modeling, but there would be other application areas where an inclusive approach would be beneficial. There are many “post-GWAS” analysis tools in the field, and they typically inherit the results of population stratification performed as a prerequisite for GWAS. The recent developments in multivariate models allow direct modeling of the continuum of genetic ancestry. It offers opportunities to develop more inclusive post-GWAS analysis methods.
Third, our work demonstrates what could be possible with methodological innovations in privacy-preserving federated learning, which, in principle, enables the sharing of individual-level data. Our study focused on a single cohort as proof of principle, and including an even larger number of individuals, possibly from multiple cohorts through the sharing of individual-level data, would further increase the power and improve predictive performance and transferability.
I am excited about all of the three future directions, and I hope many people will join our efforts.
KS: What advice do you have for trainees/young scientists?
YT: It’s a great question, partly because I am still a postdoc trainee and immensely learning from my mentors and colleagues. Here, I’d like to introduce “shu-ha-ri” (守破離), a Japanese concept of learning skills to mastery. It was a term originally used in military strategy in the 16th century, but the Japanese tea ceremony played a crucial role in the development of the idea, with substantial contributions by great tea masters, including Sen no Rikyū (1522-1591) and Fuhaku Kawakami (1719-1807). The concept was subsequently widely adopted in many disciplines, including martial arts, classical theaters, and flower arrangement in Japan. I believe some ideas around shu-ha-ri are also relevant in human genetics research.
Shu-ha-ri states learning is in three stages. In the beginning (Shu, 守), trainees observe the standard practice in the field without deviation to have a deep understanding of the traditions and the rationales behind them. Human genetics is evolving into an even more interdisciplinary field, and the importance of learning the basics cannot be overstated. Taking classes, talking to your colleagues, and consulting with your mentors help you build the foundations that prepare you for the next step.
In the subsequent stage (Ha, 破), learners explore and possibly diverge or break from the traditional techniques. In human genetics research, we can learn a lot from recent advancements in statistics, machine learning, and data analysis. The methods in those fields may not perfectly align with the standard practice in our field. Cultivating your curiosity would help you bring insights into human genetics research.
In the final stage (Ri, 離), learners have so thoroughly internalized the practice that they move beyond the original teachings, possibly establishing something entirely new as their own style. The transition to the last stage occurs naturally without intention.
As a postdoc trainee, I see myself transitioning from the first to the second stage. I would like to establish my own style in human genetics research in the future.
KS: And for fun, tell us something about your life outside of the lab.
YT: I enjoy running, swimming, and downhill skiing. I finished my first (indoor) triathlon last year and my first full marathon a few weeks ago. Outdoor physical activities help me reduce eye strain, breathe fresh air, and sometimes provide new insights.